Bioimaging techniques yield deeper insights into the molecular and cellular processes of the human body than ever before. The procedure of identifying and detecting the boundaries of specific areas of interest such as single cells within a microscopic imaging is called segmentation. In recent years, image segmentation based on deep learning has achieved great success in biomedical research. The algorithm usually learns via a large amount of “ground truth” – training data annotated by humans. This supervised learning method requires researchers to label a large amount of data. Even though unsupervised methods that can train deep learning models without human annotation exist, their performance is often less accurate than that of supervised methods – and thus not sufficient for biomedical research. That is why scientist working on the project »Smart Human-in-the-loop Segmentation« aim to develop a powerful deep learning model that is trained with a minimum amount of human effort.
Open source deep learning workflow enables more efficient image segmentation
The overall method generalises and further develops the iterative deep learning workflow of the Allen Cell and Structure Segmenter. The mentioned Segmenter is a toolkit for the 3D segmentation of intracellular structures in fluorescence microscope images, co-developed by Dr Jianxu Chen and the Allen Institute for Cell Science. The first step of the »Smart Human-in-the-loop Segmentation« is the development of effective strategies to automatically select representative samples for the initial curation or annotation based on the project team’s previous work on suggestive annotation, a deep active learning framework that combines a fully convolutional network with active learning. Next, a few samples will be selected for a rough manual annotation by the researchers. These are used for the initial training of the algorithm for the segmentation of images, which will then be applied to all images in the data set. After that, a number of images will be revised manually and entered into the workflow to train the algorithm.
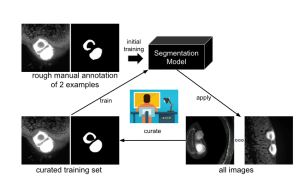
Overview of the human-in-the- loop segmentation workflow for the automated analysis of the volume of B cell follicles and T cell zone in a Peyer’s patch, a grouping of lymphoid follicles in the mucus membrane of the small intestine. The images on the left are the raw light sheet fluorescence microscopy images and the ones on the right are the images after segmentation.
© ISAS / Jianxu Chen
For each iteration, the researchers are going to develop methods to enable the deep learning model to automatically detect potential errors that will then initiate human curation. Finally, effective active learning and continual learning methods will be developed to avoid so called catastrophic forgetting. Meaning the drastic loss of previously learned information after being trained on a new task, in the iterative deep learning workflow. This enables accurate image segmentation with a low amount of human intervention. Eventually, the developed algorithms will be open source and made available as a graphical user interface tool for the broader biomedical image analysis community.
Share
Select publications
Journal of Neuroinflammation, Vol. 22, No. 1, 2025
Christ R, Siemes D, Zhao S, Widera L, Spangenberg P, Lill J, Thiebes S, Bottek J, Borgards L, Pinho AG, Silva NA, Monteiro S, Jorch SK, Gunzer M,…
Inhibition of tumour necrosis factor alpha by Etanercept attenuates Shiga toxin-induced brain pathology
https://doi.org/10.1186/s12974-025-03356-z
Biomedizinische Technik / Biomedical engineering, Vol. 70, No. 1, 2024, P. 71-90
Li J, Zhou Z, Yang J, Pepe A, Gsaxner C, Luijten G, Qu C, Zhang T, Chen X, Li W, Wodzinski M, Friedrich P, Xie K, Jin Y, Ambigapathy N, Nasca E,…
MedShapeNet - a large-scale dataset of 3D medical shapes for computer vision.
https://doi.org/10.1515/bmt-2024-0396
Computational Mechanics, Vol. 2025, 2025
Shi R, Yang H, Chen J, Hackl K, Avril S, He Y.
Deep learning without stress data on the discovery of multi-regional hyperelastic properties
https://doi.org/10.1007/s00466-024-02591-0
Biological Imaging, Vol. 4, 2024, P. e16
Zhou Y, Sollmann J, Chen J.
Deep-learning-based image compression for microscopy images: An empirical study
https://doi.org/10.1017/S2633903X24000151
JMIR Serious Games, Vol. 12, 2024, P. e52785
Egger J, Gsaxner C, Luijten G, Chen J, Chen X, Bian J, Kleesiek J, Puladi B.
Is the Apple Vision Pro the Ultimate Display? A First Perspective and Survey on Entering the Wonderland of Precision Medicine
https://doi.org/10.2196/52785
Nature Methods, Vol. 21, No. 8, 2024, P. 1390-1393
Zhang S, Dai G, Huang T, Chen J.
Multimodal large language models for bioimage analysis
https://doi.org/10.1038/s41592-024-02334-2
Journal of Cerebral Blood Flow and Metabolism, Vol. 45, No. 1, 2024, P. 171-186
Hagemann N, Qi Y, Mohamud Yusuf A, Li A, Zhang X, Spangenberg P, Squire A, Doeppner TR, Jin F, Zhao S, Chen J, Mosig A, Gunzer M, Hermann DM.
Arterial specification precedes microvascular restitution in the peri-infarct cortex that is driven by small microvessels
https://doi.org/10.1177/0271678X241270407
Dagstuhl Reports, Vol. 14, No. 1, 2024, P. 90-107
Chen J, Jug F, Rafelski S, Zhang S.
The Emerging Issues in Bioimaging AI Publications and Research (Dagstuhl Seminar 24042)
https://doi.org/10.4230/DagRep.14.1.90
Journal of Imaging Informatics in Medicine, Vol. 38, No. 1, 2024, P. 646-655
Krieger K, Egger J, Kleesiek J, Gunzer M, Chen J.
Multisensory Extended Reality Applications Offer Benefits for Volumetric Biomedical Image Analysis in Research and Medicine
https://doi.org/10.1007/s10278-024-01094-x
2024 IEEE International Symposium on Biomedical Imaging (ISBI), 2024
Zhou Y, Zhao S, Sonneck J, Chen J.
2D Label-Free Prediction of Multiple Organelles Across Different Transmitted-Light Microscopy Images with Bag-of-Experts
https://doi.org/10.1109/ISBI56570.2024.10635298
2024 IEEE International Symposium on Biomedical Imaging (ISBI), 2024
Yao J, Hagemann N, Xiong Q, Chen J, Hermann DM, Chen C.
Topological Analysis of Mouse Brain Vasculature via 3d Light-Sheet Microscopy Images
https://doi.org/10.1109/ISBI56570.2024.10635226
Nature Cardiovascular Research, Vol. 3, No. 5, 2024, P. 525-540
Tuz AA, Ghosh S, Karsch L, Ttoouli D, Sata SP, Ulusoy ?, Kraus A, Hoerenbaum N, Wolf J, Lohmann S, Zwirnlein F, Kaygusuz V, Lakovic V, Tummes H, Beer…
Stroke and myocardial infarction induce neutrophil extracellular trap release disrupting lymphoid organ structure and immunoglobulin secretion
https://doi.org/10.1038/s44161-024-00462-8
Nature Methods, Vol. 21, No. 2, 2024, P. 182-194
Reinke A, Tizabi MD, Baumgartner M, Eisenmann M, Heckmann-Nötzel D, Kavur AE, Rädsch T, Sudre CH, Acion L, Antonelli M, Arbel T, Bakas S, Benis A,…
Understanding metric-related pitfalls in image analysis validation
https://doi.org/10.1038/s41592-023-02150-0
GigaScience, 2024
Sonneck J, Zhou Y, Chen J.
MMV_Im2Im: an open-source microscopy machine vision toolbox for image-to-image transformation
https://doi.org/10.1093/gigascience/giad120
Nature Methods, Vol. 21, No. 3, 2024, P. 368-369
Zhou Y, Cao J, Sonneck J, Banerjee S, Dörr S, Grüneboom A, Lorenz K, Zhang S, Chen J.
EfficientBioAI: making bioimaging AI models efficient in energy and latency
https://doi.org/10.1038/s41592-024-02167-z
Nature Communications, Vol. 14, 2023, P. 1-13
Cibir Z, Hassel J, Sonneck J, Kowitz L, Beer A, Kraus A, Hallekamp G, Rosenkranz M, Raffelberg P, Olfen S, Smilowski K, Burkard R, Helfrich I, Tuz…
ComplexEye:a multi-lens array microscope for high-throughput embedded immune cell migration analysis
https://doi.org/10.1038/s41467-023-43765-3
Nature Machine Intelligence, Vol. 5, 2023, P. 687-698
Jia Z, Chen J, Xu X, Kheir J, Hu J, Xiao H, Peng S, Hu X, Chen D, Shi Y.
The importance of resource awareness in artificial intelligence for healthcare
https://doi.org/10.1038/s42256-023-00670-0
Nature Methods, Vol. 20, 2023, P. 968-970
Chen J, Viana M, Rafelski S.
When seeing is not believing: application-appropriate validation matters for quantitative bioimage analysis
https://doi.org/10.1038/s41592-023-01881-4
Cell Reports Methods, Vol. 3, No. 3, 2023, P. 100436
Spangenberg P, Hagemann N, Squire A, Förster N, Krauß SD, Qi Y, Mohamud Yusuf A, Wang J, Grüneboom A, Kowitz L, Korste S, Totzeck M, Cibir Z, Tuz AA,…
Rapid and fully automated blood vasculature analysis in 3D light-sheet image volumes of different organs
https://doi.org/10.1016/j.crmeth.2023.100436
IEEE Transactions on Medical Imaging, Vol. 41, No. 10, 2022, P. 2582-2597
Liang P, Zhang Y, Ding Y, Chen J, Madukoma CS, Weninger T, Shrout JD, Chen DZ.
H-EMD: A Hierarchical Earth Mover's Distance Method for Instance Segmentation
https://doi.org/10.1109/TMI.2022.3169449
Immunological Reviews, Vol. 306, No. 1, 2021, P. 293-303
Grüneboom A, Aust O, Cibir Z, Weber F, Hermann DM, Gunzer M.
Imaging innate immunity
https://doi.org/10.1111/imr.13048