Determining the cellular movement, alignment and growth of cells plays a decisive role in understanding the mechanisms of health and disease. That is why the ability to track cells in a timelapse of two- or three dimensional microscopy images plays an important part in biomedical research: It can provide important insights into the development, for example, of different forms of cancer. In the past decade, several algorithms and tools have been developed for cell tracking. However, biomedical researchers still struggle with extracting the relevant information from their microscopy timelapse data. The main reason for this is that there is no one-size-fits-all solution to handle various kinds of timelapses with, for example, different imaging settings, cell types, motion types or analysis needs. That is why the research project »Cell Tracking in Microscopy Images« aims to develop new sophisticated cell detection and tracking algorithms to tackle some of the most challenging tracking problems.
Tracking problems on which previous methods fail to obtain satisfactory results are what scientists want to change with the before mentioned project. According to them, for example the tracking process can be made extremely difficult due to overlap or clumping of the cells. Moreover, the motion of cells may vary significantly in different settings, for instance under different drug conditions. Because of this, the tracking algorithm needs to be general enough to track different possible motion patterns. The researchers are going to tackle these challenges using state-of-the-art deep learning methods.
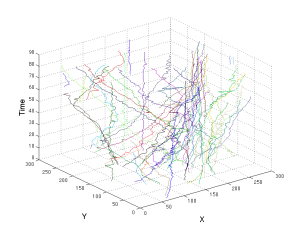
A visual representation of the trajectory of cells in space over time. Each coloured trajectory stands for a different cell. The x and y axes denote the spatial position of the cells in an image.
© ISAS / Jianxu Chen
Open source access to human-in-the-loop cell tracking via napari plugin
To make the improved analysis of microscopic images freely available to scientists worldwide, the project team is developing a human-in-the-loop cell tracking plugin for the image analysis platform napari. Conventional tools are mostly focusing on cell segmentation and tracking algorithms that are fully automated. However, in a lot of situations, the results from the fully automated algorithms do not provide satisfactory results. That is why the researcher’s goal is to bring the human back into the data analysis loop with a handy graphic user interface tool. The aim is to develop automated cell tracking algorithms that can yield the most accurate results possible, even for challenging tasks. At the same time, their cell tracking solution aims at enabling biomedical scientist to intervene in the automated analysis, correct possible errors and curate the results according to the desired accuracy. Three modules — segmentation, tracking, and analysis — will make up the "Human-in-the-loop Cell Tracking" software. Using artificial intelligence, the plugin has the objective to optimise the results of the napari image analysis and use machine learning to train the algorithm with data provided by humans.