Dortmund, 20th December 2023
Centuries ago, the invention of the microscope opened a window to a tiny, previously invisible world. Since then, it is not only the quality of images that has changed dramatically, but also the way in which we interpret them. Instead of just optically revealing the depths of the smallest structures, we can now translate biological processes into precise figures using modern image analysis methods. But for this translation to succeed, all researchers must first ask themselves the same questions: What do we want to measure? How precise do these measurements need to be so that we can interpret them in a meaningful way?
Modern microscopes generate enormous amounts of data. A light sheet fluorescence microscope at ISAS, for example, produces an average of well over 500 high-resolution images per sample. Handling this amount of data and then efficiently analysing it requires innovative approaches to data analysis. Specialised software or image analysis methods find use here, with the increasing help of artificial intelligence (AI). However, there is no such thing as a universally applicable approach to image analysis. "Just as there is no 'one size fits all' microscope, different research projects require customised image analysis workflows or software tailored to the specific requirements", explains Dr Jianxu Chen. He leads the AMBIOM – Analysis of Microscopic BIOMedical Images junior research group at ISAS, which is working on these types of AI-based biomedical image analysis algorithms.
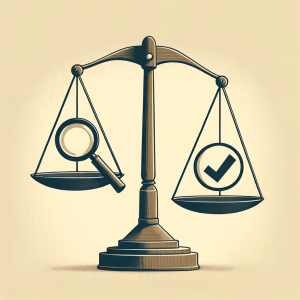
The checklist is about the balance between analysis and accuracy. This image was created by Dr Jianxu Chen (AMBIOM) on December 13, 2023, using ChatGPT, model "DALL-E". See end of page for details including prompt.
© ChatGPT
A checklist to validate bioimage analysis results
When analysing images, the challenge is to ensure that the findings obtained are not only quantitatively or statistically accurate, but also biologically meaningful to the research question. "To achieve this, researchers should fine-tune the experimental design, the details of the microscopic imaging and the target metrics of the image analysis from the start", advises Chen. Together with Dr Susanne Rafelski and Dr Matheus Viana from the Allen Institute for Cell Science in the US, he recently published some key considerations for researchers in the journal Nature Methods (see info box). Researchers can utilise them as a type of checklist when considering how to analyse their image data.
-
Basics
-
Should the measurements be relative or absolute?
Which measurements or variables are required?
Do you want to do Segmentation, deep learning prediction or do you have a specific quantification goal?
-
-
Boundaries
-
What experimental factors need to be considered?
Which microscope types and settings are suitable?
What are the limits of resolution?
-
-
Validation
-
Relative or absolute validation?
Is the goal a one-off or a robust, scalable result?
Should the accuracy apply to the entire image or specific features?
Qualitative or quantitative error bars?
-
-
Time and Effort
-
Time and effort
What is the best approach for the analysis?
How much time and effort should the analysis cost?
-
Just how good is good enough?
A key step on the way to a biologically valid image analysis is the question of validation, meaning how accurate and precise the measurements should be. "It is important that the validation is application-oriented and geared towards the specific objectives of the research", emphasises Chen. In practice, this means that researchers need to consider beforehand how accurate their measurements should be and how many errors they can tolerate. For example, if they want to measure cell parts like the nucleus or mitochondria, they could ask themselves the question: Do the exact volumes of the organelles matter, or is it more about comparing different volumes? In the latter case, the exact volume may not play a major role, but for the comparison, however, the computer programmes used must perform all the more consistently in different situations.
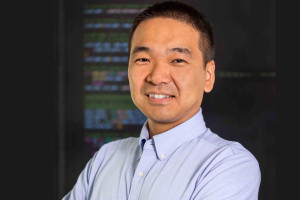
Dr Jianxu Chen
© ISAS / Hannes Woidich
Constant adaptation – with the help of AI
However, not even the best image recognition algorithms always work equally well if, for example, the shape of the organelles changes just slightly. Researchers therefore have to regularly check and validate their measurements, adjust individual parameters or, at worst, even use a completely different programme. "If such adjustments are not taken into account, this can lead to fundamental misinterpretations of the biological results", warns Chen. To him, one solution is deep learning, a form of machine learning that can automatically extract patterns and features from large amounts of data. His research group has already developed a plug-in based on deep learning for the segmentation (see infobox) of immune cells. In the future, deep learning could help researchers to automatically recognise drops in performance in their image analysis algorithms during validation.
Segmentation
In biomedical research, it can be helpful to recognise and differentiate between different structures such as cells in an image. This process is called segmentation. For a long time, it was common practice to go through the images individually and annotate (assign) them by hand. However, as data volumes and image sizes increase, researchers are increasingly turning to deep learning-based programmes. These are not only significantly faster, but also much more objective and accurate. Nevertheless, manual annotations often still serve as a training basis for these deep learning algorithms. Compared to models trained with biological ground truth, models trained with manual annotations have an error rate of over 30 per cent (see Sonneck 2023). By biological ground truth, AI experts mean the optimal approach to objective reality, which is difficult to determine with a single analysis. Instead of using manual annotations, experts determine the biological ground truth using experimental computer-aided analyses.
The future of image analysis is interdisciplinary
But Chen and his colleagues are not just interested in finding better ways to analyse images. They are keen to ensure that their methods can be understood and applied in both biology and computer technology. "We need more interdisciplinary collaboration between researchers in the lab and AI experts, preferably early on in each of their training", Chen urges. For those who cannot wait to get started, but do not have a computer vision soecialist nearby, Chen recommends online learning materials such as The Bioimage Guide or Resources via the German Network for Bioinformatics Infrastructure – de.NBI. There is also a forum at the online platform Github for specific questions. “Collaboration is the only way that quantitative microscopy image analysis can really evolve”, Chen concludes.
The MSCoreSys associated junior research group AMBIOM – Analysis of Microscopic BiOMedical Images is funded by the Federal Ministry of Education and Research (Bundesministerium für Bildung und Forschung, BMBF) under the funding reference 161L0272.
About the illustration: Prompt = „I am creating a picture illustration for my article with name “The Art of Balancing: Accuracy in Image Analysis”. It is a press article about how to do bioimage analysis validation. Could you help make an illustration, as normal as possible, and as simple as possible?” ChatGPT only provides the following information about the image: „Here is the illustration representing the concept of „The Art of Balancing: Accuracy in Image Analysis” for your article. It features a balance scale with a magnifying glass on one side and a checkmark on the other, symbolizing the equilibrium between analysis and accuracy in bioimage validation.”