Dortmund, 23rd May 2024
Artificial intelligence (AI) has evolved into an indispensable health research tool in recent years, with researchers increasingly relying on machine learning (ML) to analyse the huge data sets involved. This sub-field of AI enables computers to learn from data and recognise patterns within it, allowing researchers to better understand complex relationships, such as those that exist between courses of disease and symptoms. However, clinical data is often multi-layered. Variable and interconnected data points, such as results from a series of blood tests, quickly push standard ML algorithms to their limits. That is why researchers from ISAS, the Otto von Guericke University of Magdeburg, Bielefeld University and the German Centre for Higher Education Research and Science Studies in Hanover, together with cooperation partners from the University of Leipzig Medical Centre and Greifswald University Hospital, are turning to an alternative ML method. Taking a model for predicting sepsis (blood poisoning) as an example, the team was able to show that not only the data itself, but also the connections between the data points provide important information to facilitate early diagnosis.
Clinical practice is often a race against time. So, to be able to treat illnesses appropriately, it is essential to diagnose them early. This is exactly the case with sepsis (see info box). This life-threatening infection often progresses so rapidly that the risk of the patient dying increases by around eight percent with every hour that it goes untreated1. But in its early stages, imminent sepsis is frequently hard to detect. If sepsis is suspected, doctors can treat it initially with a broad-spectrum antibiotic, but to diagnose it specifically using a bacterial culture or by evaluating symptoms takes time. By the time the medical experts are certain which bacteria they need to treat and how, they are often battling what is already an advanced and difficult-to-treat inflammation.
SEPSIS
Sepsis, also known as blood poisoning, is a deadly disease that costs more lives every year than breast, prostate and bowel cancer combined2. The disease is caused by the immune system failing to contain a localised infection and allowing messengers and toxins to spread through the bloodstream. The body responds by sending out leukocytes (white blood cells) to fight the pathogens (causative organisms). This can cause the blood vessels to expand. Since the patient's blood pressure then falls, vital organs such as the lungs, kidneys or heart are no longer being supplied with enough oxygen via the blood and, in serious cases, may fail. The patient enters septic shock, which means their life is in acute danger.
Machine learning to facilitate early diagnosis
In future, machine learning (see info box) could help doctors to diagnose time-critical diseases at an early stage: "Using AI, we are able to look at blood counts and predict which patients may be at risk of developing sepsis, for example," says Prof. Dr Robert Heyer, Head of the Multidimensional Omics Data Analysis junior research group at ISAS. He goes on to say that corresponding models do already exist: "But they reach their limits when asked to take complex time series into account." Time series are made up of sequences of data points, which are collected at regular intervals. In a hospital setting they may contain information about changes to patients' vital signs such as blood levels, heart rate or blood glucose level, for example.
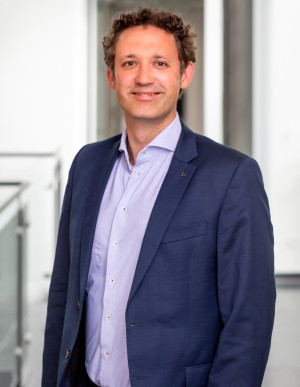
Our results underline how important data collected regularly from patients is in providing basic information for predictive models in the field of health research.
Prof. Dr Robert Heyer
The team of interdisciplinary researchers put a specific type of algorithm, called a graph neural network (GNN), to the test. These networks are particularly well suited to analysing data organised in the form of graphs – such as time series. In a graph, the data points (nodes) are connected to one another by "edges", which represent the relationships between the nodes. This gives rise to complex network structures, in which the nodes take their own information into account as well as information about their neighbouring nodes. By following the links within the data network, GNNs decode how various factors affect one another and reveal hidden connections. "Our objective was to find out how suitable GNNs are for analysing complex clinical data and whether integrating time series improves the predictive accuracy of the models," says Daniel Walke, doctoral candidate at the Otto von Guericke University of Magdeburg and lead author of the joint pre-print (pre-release of a scientific paper that has not yet been peer reviewed).
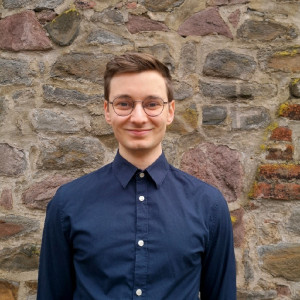
Daniel Walke is a PhD student in the Database and Software Engineering group at Otto von Guericke University Magdeburg.
© Privat.
MACHINE LEARNING
Machine learning (ML) is a discipline of artificial intelligence. With the help of ML, computers are trained to process data and previous experiences independently and adapt to them accordingly. An example of ML are artificial neural networks (ANNs), which are modelled on the human brain. They consist of artificial neurons, which are arranged in layers and connected to one another. These neurons process inputs, perform calculations and provide outputs. Training the network on sample data allows it to detect patterns and relationships so it can perform tasks such as making predictions or recognising patterns, for example. Graph neural networks (GNNs) are a particular kind of ANN. They can also take account of additional information from linked measurements, often referred to as "message passing". To make their predictions and classifications, GNNs use the structure and relationships (edges) within a graph to understand how the data points (nodes) interact with and influence one another.
Time series improve predictive power
The researchers based their work on a data set containing information about over 528,000 people, who were treated on the wards (with the exception of intensive care) at the University of Leipzig Medical Centre and Greifswald University Hospital between the years 2014 and 2021. Some of them suffered from sepsis during their time in hospital, while others did not. The researchers used the extensive data from Leipzig to first train their GNNs to retrospectively predict the probability of sepsis. Applying the GNNs to the data set from Leipzig, and another from Greifswald, showed similar results to those achieved using conventional ML algorithms and other types of neural network. However, applying GNNs to time series data, which incorporate test results for the same patients, gave significantly better results. Unlike previously, when similar test results from various patients were linked to one another, the nodes now represent full blood counts from just one person at different points in time. The researchers used the AUROC (Area Under the Receiver Operating Characteristic) curve to measure the reliability of the predictions. The closer the value is to 1, the better the model is performing. Heyer and his team were able to improve the AUROC values from under 0.88 to over 0.95 by integrating time series. "The fact that time series have such a big impact on the reliability of the predictions underlines how important data collected regularly from patients is in providing basic information for predictive models in the field of health research," sums up Heyer.
Not a black box: medicine needs transparency
At the moment, how well GNNs can really be integrated into everyday medicine is still largely untested. Another challenge is this: "GNNs and other complex machine learning algorithms (e.g. XGBoost) are often treated as black-boxes limiting their interpretability and transparency which is essential for medical applications," write Heyer and his fellow researchers in their paper. It was therefore important for the authors to understand exactly what the algorithms were basing their predictions on. "That's why we didn't just leave it as a black box. We tried to find out what the algorithms had learnt from the patient data. We wanted to know what factors were behind their predictions," says Heyer. When it comes to sepsis, the analysis shows that, besides the varying number of white blood cells, the key factor is their interactions with other types of blood cell.
Advanced ML tools could potentially save countless lives – not only from sepsis, but from other diseases too. In future, GNN analyses of blood count data could help to diagnose thrombosis or leukaemia, for example.
Article Recommendation
Walke, D., Steinbach, D., Gibb, S., Kaiser, T., Saake, G., Ahrens, P., Broneske, D., Heyer, R.
(2023). Edges are all you need: Potential of Medical Time Series Analysis with Graph Neural Networks. PREPRINT (Version 1) available at Research Square: https://doi.org/10.21203/rs.3.rs-3573549/v1.
1 https://www.ncbi.nlm.nih.gov/pmc/articles/PMC8210984/
2 https://www.england.nhs.uk/blog/beating-sepsis-with-early-detection-and-prompt-treatment/
(Cheyenne Peters/ Ute Eberle)