Dortmund, 31st October 2024
Prof Dr Yiyu Shi holds a professorship in computer science and engineering at the University of Notre Dame, U.S., and is Director of their Sustainable Computing Laboratory. His research focuses on deep learning, hardware acceleration, and medical applications. Since he is also a visiting scientist at Boston Children’s Hospital, the primary paediatric programme of Harvard Medical School, Shi is very familiar with the interface between science and clinic. The 40-year-old researcher is the corresponding author of the Perspective article in Nature Machine Intelligence. A report on the article, whose authors include ISAS researcher Dr. Jianxu Chen, was published here on ISAS Kompakt on October 21.
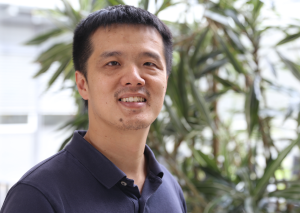
Prof Dr Yiyu Shi knows the interface between science and the clinic particularly well. Shi teaches at the University of Notre Dame (USA) and conducts research at Boston Children's Hospital.
© ISAS / Hannes Woidich
Article Recommendation
Jia, Z., Chen, J., Xu, X., Kheir, J., Hu, J., Xiao, H., Peng, S., Hu, X.S., Chen, D., Shi, Y.
(2023) The importance of resource awareness in artificial intelligence for healthcare. Nature Machine Intelligence (5): 687-698. https://doi.org/10.1038/s42256-023-00670-0
Professor Shi, do you think that when it comes to energy costs, hospitals may need to not only concentrate on how to reduce energy costs for the buildings and medical equipment like MRIs, but also think about how they handle their data?
Shi: Yes, of course. Energy is always a problem, because many hospitals have to store their data locally. And this can become more of a problem in the future as not every hospital has all the facility that would be necessary for a full-scale data centre. In terms of using the data, you want to analyse them or apply state-of-the-art machine learning models or other advanced techniques. But these consume a big amount of energy as well. That is why we are looking at the sustainability of these kind of AI techniques. We want to help reduce the energy footprint for the data storage and analysis at the same time.
When thinking of the technological aspects, why do you and your co-authors make a case for recycling and thinking small by using algorithms designed for tiny networks or reducing the size of your models?
Shi: It takes a lot of resources to train a model from scratch. I understand the idea because every individual hospital has its own specific data. There is a need to work out a personalised model for each individual centre or hospital, but I think it is more or less a waste to train every model from scratch. You can start from a pre-trained model that has certain knowledge of a particular problem and then fine tune it, based on the specific needs. This would save a lot of resources. So yes, to some extent we can recycle. The size of the model also matters because even if you take a model that has already been trained for a particular hospital, every time you have to run inference on that part, this will lead to a big energy consumption. A smaller model would not necessarily maintain the same performance as regular sized model, but the smaller it is, the better it is in terms of resource consumption. To put it in a nutshell: It is best to find a model that is just big enough to meet the need and at the same time not too big to overconsume your resources.
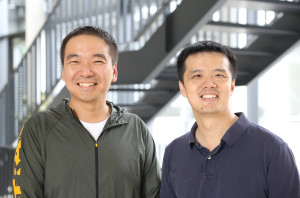
Dr Jianxu Chen (left) from the ISAS research group AMBIOM and Prof Dr Yiyu Shi from the University of Notre Dame (USA) are among the authors of the publication in Nature Machine Intelligence. The photo was taken during a guest lecture at ISAS.
© ISAS / Hannes Woidich
You state that right now, the network infrastructure in hospitals and other healthcare facilities is not designed to handle massive amounts of data that, for example, deep learning requires. Regarding training deep neural networks collaboratively, what would it take to be able to do this?
Shi: For example, to share the data in order to train a model, you need to remove all the personal patient information, which is still quite a lot of work. However, there are public domain datasets available. It would make sense to pre-train a model on whatever public domain data set can be obtained. And then start from that model to adapt it to the individual data from a particular hospital. However, at least in the U.S., it is relatively easy to work together and share data if the hospitals belong to the same management. When it comes to hospitals across different states, it is much more difficult due to privacy and security concerns. My and my co-authors’ vision is that there will be ways possible – not just from a scientific perspective, but rather from a public, policymakers’ perspective – to have an umbrella agreement that allows easier data sharing or at least some kind of experiments.
You advocate for more medical domain experts in order to be able to keep up with the increasing number of medical images needed for training the AI models, and also to assure a good training. What benefit do AI models have over telemedicine, where you still need a doctor to “see” the patient remotely and make the diagnosis?
Shi: Telemedicine makes healthcare accessible to a broader population, especially in rural areas. Nonetheless, you still have a limited number of physicians with limited time to participate. So there is a restriction of how much the population can really benefit from telemedicine. With the help of AI, there is basically an unlimited availability for at least some screenings for simple healthcare problems. Me and my team at Boston Children’s Hospital are working on a diagnosis app for common skin diseases. Our goal is that using AI models, we can – with the help of medical expertise – train the app at least to tell patients with high accuracy whether they should see a doctor immediately or whether they are dealing with symptoms that are not urgent and can wait for a scheduled appointment. Everyone with a mobile phone will have access to the app.
(The interview was conducted by Sara Rebein.)